Preventive maintenance is a cornerstone of asset management, and for many organizations, integrating AI into their maintenance programs seems like a no-brainer. Yet, while businesses race to harness AI’s analytical power, they’re often overlooking a critical factor: their data. In fact, many teams are falling into a common trap—focusing on AI’s potential without addressing the data quality issues that undermine its effectiveness.
Here’s the reality: bad data leads to bad analysis, no matter how advanced your AI is. And most teams aren’t just grappling with bad data—they’re stuck in inefficient processes that perpetuate the problem. Even worse, they’re missing out on the most impactful way to use AI: improving the very processes that generate their data.
The trap: all eyes on analysis, none on collection
It’s easy to get swept up in the possibilities of AI analysis. Predicting equipment failures, optimizing maintenance schedules, identifying inefficiencies—the potential benefits are enormous. But here’s the catch: AI is only as good as the data it analyzes. If the data is incomplete, inconsistent, or outright wrong, the insights will be flawed, or worse, useless.
Why is this happening? Because organizations spend their innovation budgets on analyzing the data they have instead of ensuring that data is high-quality to begin with. The result? Garbage in, garbage out.
The root cause lies in the field—where data is actually collected. Most teams rely on outdated, cumbersome tools for data capture. This creates a vicious cycle of poor data collection leading to poor data quality, which undermines AI’s impact.
The field problem: inefficient tools, inefficient processes
Data collection in the field is notoriously challenging. Many teams rely on bolt-on apps that are retrofitted to asset management programs or custom-built solutions that are difficult and expensive to maintain. These systems often fall out of date, leading fieldworkers to revert to paper forms or makeshift spreadsheets.
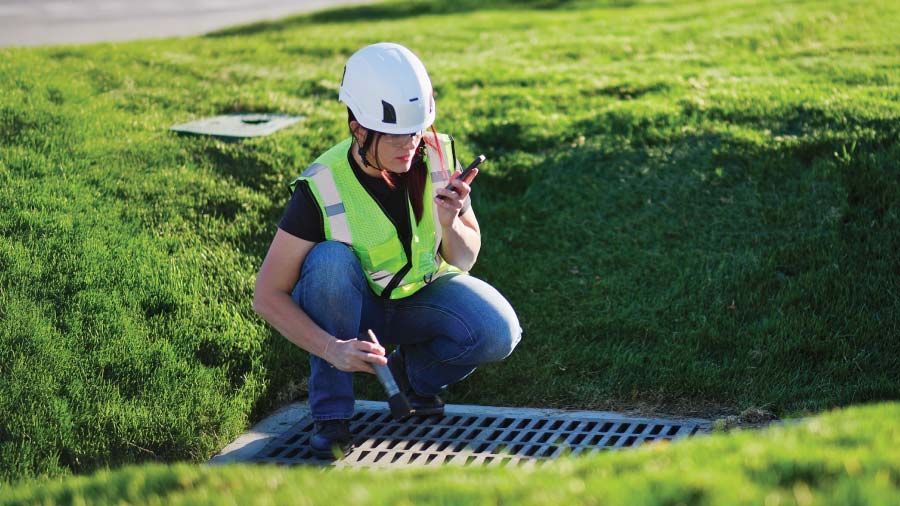
These inefficiencies in field data collection have cascading effects:
- High costs. Inefficient tools result in higher total costs of ownership (TCO) for field data collection apps.
- Frustrated workers. Field staff spend more time on data entry, detracting from their primary responsibilities.
- Missed opportunities. Poor data limits the effectiveness of AI analysis, reducing the return on investments in AI.
The shift: Focus AI innovation where it matters most
To break this cycle, organizations need to rethink how they’re deploying AI. Instead of focusing solely on analysis, they should prioritize improving data collection processes in the field. This shift has two major advantages:
- Maximizing labor efficiency
Field staff are often highly trained professionals with deep knowledge of the assets they maintain. Yet they spend a significant portion of their time on clerical tasks like data entry. By streamlining these tasks with AI-powered tools, organizations can free up their field teams to focus on higher-value work, reducing costs and improving productivity. - Enabling better data for analysis
High-quality data doesn’t happen by accident. By equipping fieldworkers with AI tools that simplify and standardize data capture, organizations can ensure that the information feeding into their AI systems is accurate, consistent, and actionable.
How AI can transform field data collection
So, what does this look like in practice? Below are two high-impact ways AI can revolutionize preventive maintenance field data collection.
Voice dictation for seamless reporting
Field teams often need to document equipment status, observations, and maintenance actions. Traditionally, this means typing on a mobile device or jotting notes on paper—both time-consuming and error-prone. AI-powered voice dictation allows workers to capture detailed, accurate notes simply by speaking. This eliminates the need for manual data entry, speeds up reporting, and reduces errors.
Automated nameplate data capture
Equipment nameplates contain critical information, from model numbers to operational specifications. Manually recording this data is tedious and prone to mistakes. AI can streamline this process by capturing and digitizing nameplate data using image recognition. This not only saves time but also ensures accuracy, providing a reliable foundation for AI-driven analysis.
The payoff: Why field AI comes first
Investing in AI for field data collection isn’t just about making life easier for field teams. It’s about laying the groundwork for success in every other AI initiative. High-quality data collected efficiently in the field feeds directly into advanced AI tools for analysis, enabling organizations to:
- Predict equipment failures with greater accuracy
- Optimize maintenance schedules based on real-world conditions
- Reduce costs by preventing downtime and extending asset life
In other words, the benefits of AI don’t start with analysis; they start in the field. By focusing on field AI, organizations can unlock the full potential of their PM programs.
Rethink your approach to AI in preventive maintenance
Preventive maintenance starts with the right tools and processes for data collection. By equipping your field teams with AI-powered tools, you can not only streamline operations but also ensure your data is ready for advanced analysis. This foundational step is key to making every AI initiative a success.
Of course, none of this obviates the need for AI in other aspects of preventive maintenance. It’s not an either-or choice but a complementary “both” approach that compounds the benefits of AI. By addressing data collection and analysis in tandem, organizations can achieve far greater impact across their maintenance programs.
If you’re ready to see how AI can transform your field data collection and maintenance strategies, contact us for a demo today. We’d love to show you how Fulcrum can help you build a better foundation for your preventive maintenance program.